EPOCH Tutorials - Preparing and Cleaning Data for Historical Research with Microsoft Excel
- EPOCH
- Jun 1, 2024
- 8 min read
Edward Moore | Lancaster University
Preparing and cleaning data is often a time-intensive and, frankly, rather dull process for most. As historians, our data is often just as messy as the history we study - if not more so. Data from the past can range from our primary source to databases containing archaeological finds. The methods of cleaning data are fairly standard regardless of what form your data is in. Whether your data is a spreadsheet or a written primary source, you want to remove what I’ll call ‘clutter’; things such as additional spaces, editorial comments, and footnotes all add clutter to your data that makes its use elsewhere difficult and fiddly. You also want to separate individual pieces of data so that they can be found and used more easily by external software. Finally, you want some degree of standardisation in your data to make comparisons easy, make useful visualisations, and make your data easier to navigate and filter.
This tutorial will make cleaning data held in spreadsheets more manageable and less daunting. It aims to share practical guidance on how to speed up the process and showcase several methods for data cleaning. Together, we shall use some sample data from Scotland’s National Record of the Historic Environment Database ‘Canmore’ to practice data cleaning and extract more useful information from the results.
Data Cleaning and Visualisation using Data from the 'Canmore' Database
The Canmore database, easily accessible here, allows for an easy data collection phase, but the data contained within its records needs cleaning. In this example, we will utilise Canmore's records on 'Pictish' items in Scotland, but the process is is universal to any data extracted from Canmore and similar databases (such as those held by PAS, Historic England, Cadw, etc.), as well as most data held in a spreadsheet format. This tutorial will use Microsoft Excel, however, most major spreadsheet software has tools equivalent to those used here.
Downloading from Canmore
Downloading data from Canmore is relatively straightforward, using their Site Search page. This allows you to search for specific types of historical sites in Scotland. We are simply going to imput 'Pictish' as the keyword, and leave everything else blank (but the country should be left as the default 'Scotland Only' for this exercise). At the time of writing, this search returns 626 results. These results can be filtered to further specifications: Classification, Council, (Historical) County, Parish, Discipline, and Form. At the bottom of the page will be a section labelled 'Download'; this allows you to download the data matching your section criteria. Underneath this section are two options ', CSV' and 'KML'. In most instances, you will want to download the data as a CSV so that it can be used in spreadsheet software such as Microsoft Excel.
These options are not available for searches that return over 1,000 results, in which case you will instead have to access Canmore's full data download (here) and find your results there. Beware that there are some 340,000 entries in the Canmore database, so making a series of smaller downloads and adding them to a common spreadsheet may be easier.
Cleaning Canmore Data
Cleaning Canmore data is a relatively straightforward but multistep process. This process is more or less universal to all data held in databases and stored in spreadsheets and tables (such as parish records and census data). There are three main steps:
Setting your data up as an Excel Table.
'Atomising' the SITE TYPE column into separate columns.
Removing unnecessary data and text.
Setting your data up as an Excel Table
First, you will need to convert the CSV format of the file; this involves clicking 'Save As' under the File tab of Excel, then clicking the drop-down menu that appears and ensuring it is set as 'Excel Workbook (.xlsx)'. It should go from looking like the above image to the one below. Click 'Save', and the format has been converted.


To create the Table, select all your data and press 'CTRL+T'. Alternatively, you can easily select all your data by clicking anywhere on it and pressing 'CTRL+A'.
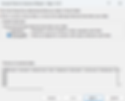
The following window will appear. Ensure that the 'My table has headers' button is checked, then press 'OK'; your data will now be stored in a table. For easier reference later, we will rename the table 'Pictish_Sites'. Do so by selecting the 'Table Design' tab when the table is selected and renaming it in the text box to the far left (as below). The use of an underscore is needed in lieu of a space.

Tables are incredibly useful and should be used in Excel almost any time that you will be manipulating data in any way. Especially if doing so through formulae, as it will automatically apply any formula in one row to every other row in that column and update them as you change the first. It also makes it easier to filter results, which can help speed up the data-cleaning process.
Atomising and Standardising Data

As seen in the table above, the SITE TYPE column contains quite a few different data points. For this data to be of use to most other software and for easier manipulation, this data will need to be atomised and then standardised. For example, most pieces of software aren’t smart enough to distinguish between a Canmore entry of ‘Enclosure’ and ‘Enclosure(S)’ despite them describing the same feature, because they are not written exactly the same.
First, we’ll extract the date information held here to create three new columns: a period range, the earliest period, and the latest period column for each site. Since we are looking for truly Pictish Items, you will want to remove any results dated to a significantly later period. I removed everything later than 'Post Medieval'.
Next, I went through and assigned the period range of each site's entry, writing it as 'Earliest Period - Latest Period'. For the 'Earliest Period' and 'Latest Period' columns, I utilised Excel's built-in functions BEFORETEXT and AFTERTEXT, respectively, to automatically return the relevant period. The formula entered into each column should look like this:

It's important to note that the delimitator should be entered as " - " including both spaces so as not to pick up any extra spaces in the returned results.
It is also important that the is_not_found cell is set as the Period Range cell so that a result is still returned even if no range is present.
As Canmore uses both 'Period Unassigned' and 'Period Unknown' as its entry for yet-to-be-dated and impossible-to-date categories, I inputted results that just had that assigned dating as 'Unknown'.
In the end, you should have something looking like this:

With the dates now separated, we can fully atomise the SITE TYPE column using Excel's 'Text to Column' tool.

Opening the 'Text to Column' tool will result in the window below appears, as a comma already separates the data from Canmore; ensure that Delimited is selected, then click next.

On the next screen, select the comma and nothing else, then click 'Next'.

Finally, ensure your data looks like the Data preview below. The destination should be in column O, but it may vary based on how your data is arranged; in any case, ensure it is placed right of the rest of your data.

The output should look like the table below. For the sake of clarity, each column was named TYPE X.

While the data is now fully atomised, it still needs a final round of cleaning. This is the most straightforward part of data cleaning and involves removing the period allocations and instances of (S) in each column. The replacement tool is the quickest way to do so. You may also wish to remove the few instances where the material type is distinguished in the column, but I left those in place.
Now that this has been done, the data is more or less ready to be used in various digital processes, including visualisation, importation to other software, and the extraction of further useful information.
Extracting Useful Information with Pivot Tables
We now have cleaned data. However, the data is still just data; to be of much further use, we need to extract information from the data. While this can be done in more specialist software, such as Geographic Information Systems, today, we will focus on extracting quantitative information from the data we have already cleaned using Excel’s Pivot Tables. While seen as a bit of a daunting ‘black box’, they are easy to set up once you know how. Go to the Table Design tab as you did for changing the table name and select 'Summarize with Pivot Table'.

The following window will appear:

The default settings should be suitable; ensure your Table name appears in the 'Table/Range' text box and New Worksheet is selected, then press 'OK', creating a new worksheet with an empty Pivot Table. The result should look as follows:

We will now use the Pivot Table to summarise several useful statistics about our now-cleaned data in preparation for various uses, such as visualisation and simple metric extraction.
First, we will get some statistics about the geographical distribution of Pictish sites in the Canmore database by selecting 'COUNCIL' and then 'CANMORE ID'. This should automatically place 'COUNCIL' in the 'Rows' box and 'CANMORE ID' in the 'Values' box.
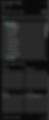
You will notice that the item that appears in the 'Values' box is 'Sum of CANMORE ID'. We want to change this to be useful. Click on the item and select 'Value Field Settings' (it should be the option at the very bottom of the list that appears).

On the window that appears, select 'Count' as how the values will be summarised. This will result in a Pivot table showing the count of each item per County Council. The Pivot Table should look as below.

Now select the pivot table, and copy and paste it into a new worksheet, ensuring to use 'Paste Values'.
For the next series of information we want to be summarised in the Pivot Table, we will place our recently created 'Earliest Period' column in the Rows box and keep 'Count of CANMORE ID' in the values box. It should look as below. Copy and paste the table's content as before.

You now have two sets of useful information to use as you wish. For example, I used the results of the first table we made to create the choropleth map below.

While there are many other means of extracting useful information from cleaned data, Pivot tables are one of the most universal methods and have the least risk of human error.
Final thoughts
Many may consider data cleaning a superfluous act, but by cleaning your data, you make it much easier to extract useful information from your wider data and use it in further digital methods, such as viewshed analysis and creating visualisations such as choropleth maps.
While Excel is not the most powerful tool for data cleaning, it is an easy option for newcomers to historical data. It provides a number of tools, such as ‘Text to Column’, which makes atomising data much easier. Excel also provides a neat package if you then want to go on and visualise, further manipulate or share your data in a straightforward manner.
Further Reading:
Christof Schöch, 'Big? Smart? Clean? Messy? Data in the Humanities?', Journal of the Digital Humanities, 2 (2013).
'Journal of Open Humanities Data', Ubiquity Press, (2024) <https://openhumanitiesdata.metajnl.com/> [Accessed 27 May 2024].
Edward is a third-year PhD student at Lancaster University. His research aims to understand the various factors that influence the selection of sites for early medieval stone sculptures in western Northumbria, looking at visibility, the correlation and similarities of these sites in their wider landscapes with other features in those landscapes.